Data Science Boot Camp
Spring 2023
May 9, 2023
-
Jun 8, 2023
I'm a paragraph. Click here to add your own text and edit me. It's easy.
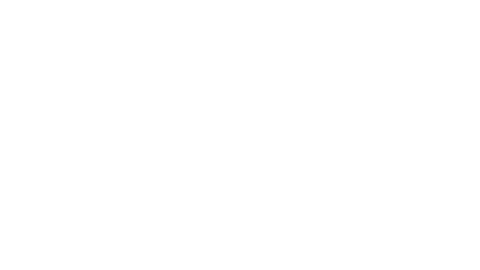
Checking your registration status...
You are registered for this program.
Registration Deadlines
Mar 16, 2023
-
Academics from Member Institutions/Departments
Mar 16, 2023
-
Academics from Non-Member Institutions paying the $500 membership fee
Jan 16, 2023
-
Academics from Non-Member Institutions applying for Corporate Sponsored Fellowships
Category
Launch
Overview
The Erdős Institute's signature Data Science Boot Camp has been running since May 2018 thanks to the generous support of our sponsors, members, and partners. Due to its popularity, we now offer our boot camp online twice per year in two different formats: a 1-month long intensive boot camp each May and a semester long version each Fall.

#slack-channel
Organizers, Instructors, and Advisors
Objectives
The goal of our Data Science Boot Camp is to provide you with the skills and mentorship necessary to produce a portfolio worthy data science/machine learning project while also providing you with valuable career development support and connecting you with potential employers.
Those who successfully complete a team project will receive a digital certificate of completion with a sharable URL.
Project Examples
TEAM
Aware NLP Project III
Mohammad Nooranidoost, Baian Liu, Craig Franze, Mustafa Anıl Tokmak, Himanshu Raj, Peter Williams




This project involves the investigation and evaluation of different methodologies for retrieval for use in RAG (Retrieval-Augmented Generation) systems. In particular, this project investigates retrieval quality for information downloaded from employee subreddits. We investigated the impacts of using clustering, multi-vector indexing, and multi-querying in advanced retrieval methodologies against baseline naive retrieval.
First Steps/Prerequisites
Program Content
I'm a paragraph. Click here to add your own text and edit me. It's easy.
Textbook/Notes
Project/Homework Instructions
I'm a paragraph. Click here to add your own text and edit me. It's easy.
Schedule
Click on any date for more details
Matt Osborne Office Hour
May 3, 2023 at 8:00:00 PM
EVENT
Data Science Boot Camp AM Problem Session 1
May 9, 2023 at 2:00:00 PM
EVENT
Data Science Boot Camp AM Problem Session 2
May 10, 2023 at 2:00:00 PM
EVENT
Data Science Boot Camp AM Problem Session 3
May 11, 2023 at 2:00:00 PM
EVENT
Matt Office Hour
May 12, 2023 at 3:00:00 PM
EVENT
Data Science Boot Camp AM Problem Session 4
May 15, 2023 at 2:00:00 PM
EVENT
Data Science Boot Camp AM Problem Session 5
May 16, 2023 at 2:00:00 PM
EVENT
Data Science Boot Camp AM Problem Session 6
May 17, 2023 at 2:00:00 PM
EVENT
Data Science Boot Camp AM Problem Session 7
May 18, 2023 at 2:00:00 PM
EVENT
Matt Office Hour
May 19, 2023 at 3:00:00 PM
EVENT
Data Science Boot Camp PM Problem Session 8
May 22, 2023 at 8:00:00 PM
EVENT
Data Science Boot Camp PM Problem Session 9
May 23, 2023 at 8:00:00 PM
EVENT
Data Science Boot Camp PM Problem Session 10
May 24, 2023 at 8:00:00 PM
EVENT
Data Science Boot Camp PM Problem Session 11
May 25, 2023 at 8:00:00 PM
EVENT
Matt Office Hour
May 26, 2023 at 7:00:00 PM
EVENT
Matt Office Hour
May 31, 2023 at 6:00:00 PM
EVENT
Erdős Final Project Showcase and Commencement
June 7, 2023 at 4:00:00 PM
EVENT
Matt Osborne Office Hour
May 5, 2023 at 3:00:00 PM
EVENT
Data Science Boot Camp PM Problem Session 1
May 9, 2023 at 8:00:00 PM
EVENT
Data Science Boot Camp PM Problem Session 2
May 10, 2023 at 8:00:00 PM
EVENT
Data Science Boot Camp PM Problem Session 3
May 11, 2023 at 8:00:00 PM
EVENT
Matt Office Hour
May 12, 2023 at 7:00:00 PM
EVENT
Data Science Boot Camp PM Problem Session 4
May 15, 2023 at 8:00:00 PM
EVENT
Data Science Boot Camp PM Problem Session 5
May 16, 2023 at 8:00:00 PM
EVENT
Data Science Boot Camp PM Problem Session 6
May 17, 2023 at 8:00:00 PM
EVENT
Data Science Boot Camp PM Problem Session 7
May 18, 2023 at 8:00:00 PM
EVENT
Matt Office Hour
May 19, 2023 at 7:00:00 PM
EVENT
Data Science Boot Camp Lecture 9
May 22, 2023 at 9:30:00 PM
EVENT
Data Science Boot Camp Lecture 10
May 23, 2023 at 9:30:00 PM
EVENT
Data Science Boot Camp Lecture 11
May 24, 2023 at 9:30:00 PM
EVENT
Data Science Boot Camp Lecture 12
May 25, 2023 at 9:30:00 PM
EVENT
Matt Office Hour
May 29, 2023 at 8:00:00 PM
EVENT
Matt Office Hour
June 1, 2023 at 2:00:00 PM
EVENT
Data Science Boot Camp Lecture 1
May 8, 2023 at 9:30:00 PM
EVENT
Data Science Boot Camp Lecture 2
May 9, 2023 at 9:30:00 PM
EVENT
Data Science Boot Camp Lecture 3
May 10, 2023 at 9:30:00 PM
EVENT
Data Science Boot Camp Lecture 4
May 11, 2023 at 9:30:00 PM
EVENT
Project Pitch Day (Live on Zoom)
May 12, 2023 at 8:30:00 PM
EVENT
Data Science Boot Camp Lecture 5
May 15, 2023 at 9:30:00 PM
EVENT
Data Science Boot Camp Lecture 6
May 16, 2023 at 9:30:00 PM
EVENT
Data Science Boot Camp Lecture 7
May 17, 2023 at 9:30:00 PM
EVENT
Data Science Boot Camp Lecture 8
May 18, 2023 at 9:30:00 PM
EVENT
Data Science Boot Camp AM Problem Session 8
May 22, 2023 at 2:00:00 PM
EVENT
Data Science Boot Camp AM Problem Session 9
May 23, 2023 at 2:00:00 PM
EVENT
Data Science Boot Camp AM Problem Session 10
May 24, 2023 at 2:00:00 PM
EVENT
Data Science Boot Camp AM Problem Session 11
May 25, 2023 at 2:00:00 PM
EVENT
Matt Office Hour
May 26, 2023 at 3:00:00 PM
EVENT
Matt Office Hour
May 31, 2023 at 2:00:00 PM
EVENT
Matt Office Hour
June 1, 2023 at 8:00:00 PM
EVENT
Please check your registration email for program schedule and zoom links.
Project/Homework Deadlines
May 12, 2023
8:30 PM
Project Pitch Day (Live on Zoom)
Opportunity to meet with other Erdos Fellows and form teams and propose topics.
May 13, 2023
3:59 AM
Submit Team Proposal to Project Formation Page
If you want to propose a project, or have an idea for a project, submit it by this date.
May 15, 2023
3:59 AM
Finalized Teams with Preliminary Project Idea
Teams need to be finalized by this point. If you proposed or created a project, you must have others in your group. If you did not propose or create a project, you must join an open group.
May 20, 2023
3:59 AM
Data gathering and defining stakeholders + KPIs
Find the dataset you will be working with. Describe the dataset and the problem you are looking to solve (1 page max). List the stakeholders of the project and company key performance indicators (KPIs) (bullet points).
May 20, 2023
3:59 AM
Data cleaning + preprocessing
Look for missing values and duplicates. Basic data manipulation & preliminary feature engineering.
May 27, 2023
3:59 AM
Exploratory data analysis + visualizations [Checkpoint]
Distributions of variables, looking for outliers, etc. Descriptive statistics.
May 27, 2023
3:59 AM
Written proposal of modeling approach [Checkpoint]
Test linearity assumptions. Dimensionality reductions (if necessary). Describe your planned modeling approach, based on the exploratory data analysis from the last two weeks (< 1 page, bullet points).
Jun 2, 2023
3:59 AM
Machine learning models or equivalent [Checkpoint]
Results with visualizations and/or metrics. List of successes and pitfalls.
Jun 3, 2023
4:00 PM
Final project due
Please read the submission instructions on the link below.